Transforming banking
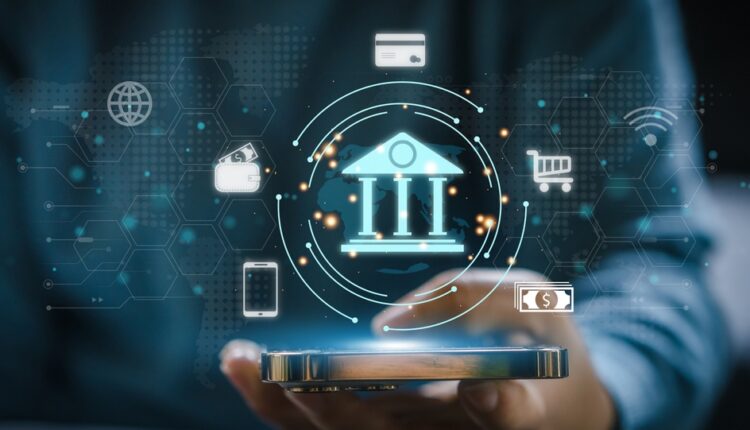
In today’s rapidly evolving banking landscape, staying ahead requires more than just innovative products and services—it demands a data-driven approach that provides real-time insights and actionable intelligence. We sat down with Bruno Ascencio, Head of Data Transformation at First Abu Dhabi Bank, to delve into how they leverage Confluent Kafka and other cutting-edge technologies to modernize legacy systems, enhance customer experiences, and bolster their data governance practices.
Can you provide more details about how FAB leverages the Confluent platform?
We are harnessing Confluent Kafka in two different ways. Firstly, to modernize our legacy systems and existing bank applications, we aimed to enhance our service without disrupting the experience for both internal and external customers. By incorporating a Kafka layer into these legacy systems, we were able to augment the existing Service Level Agreements (SLAs) or even upgrade them, all without the need to replace the core platform.
Secondly, as we integrate cloud technologies into our architecture, our focus is on deploying advanced AI features and machine learning models for risk prevention. This strategic move enables us to evaluate our customers and transactions with the ability to deploy these models in real-time or near-real-time scenarios. This is a significant shift from the traditional approach, which could take several hours or even a day for fraud detection, a delay that could have severe consequences for operations as critical as ours at FAB.
What specific benefits or outcomes have you achieved in this area?
In the context of risk prevention, our current focus is on streaming data for corporate-side transactions. This data stream includes critical information, particularly related to cross-border transactions. We are particularly interested in managing aspects such as the counterparty banks we are transacting with and the purpose of purchase or payment. These details provide valuable insights into the real-time rationale behind these transactions.
By continuously monitoring these details, we can now quickly identify any anomalies or potential red flags, enabling us to take immediate action when needed. It’s worth noting that while our successful testing and deployment efforts initially centered around corporate banking, we are now in the process of extending these capabilities to consumer banking as well.
How does real-time CRM enhance FAB’s customer engagement and service delivery, and how does the Confluent platform play a role in this?
Real-time CRM is one of the largest projects our consumer banking team is currently engaged in, which will extend into the next year. This project centers around achieving what is known as “hyper-personalization.” In our pursuit of hyper-personalization, we are placing great emphasis on contextual layers. We aim to move beyond simply providing you with the best offer, a capability our machine learning models and data scientists have already developed. Our focus now is on advancing to the next level, where real-time or micro-batching data plays a pivotal role. It’s not only about determining which offer is the best fit for you; it’s also about identifying the appropriate context for delivering this offer to you. We strive to understand the specific context in which the customer finds themselves at that precise moment. This level of personalization is often referred to as “hyper-personalization.”
Payment processing and clearing require high reliability and efficiency. How has the Confluent platform improved FAB’s payment processing operations, and what challenges has it helped address?
The Central Bank in the UAE has made NPSS (National Payment Systems Strategy) one of its top priorities. This strategic focus is central to their efforts to transition towards a cashless, real-time transaction economy. As part of this transition, every bank in the region must offer capabilities that enable customers to easily visualize and comprehend real-time transactions.
Currently, any transaction amounting to less than 50,000 AED can be processed and visualized in real time through the FAB application. This represents our initial step, serving as a stepping stone towards the seamless deployment of these NPSS within our application.
Can you share insights into the scalability and performance enhancements that FAB has experienced since implementing Confluence?
Certainly, as I mentioned earlier, Confluent is playing a dual role in assisting us. Firstly, it aids in the modernization of our legacy systems, and secondly, it adds an additional layer of intelligence. While our journey towards cloud migration and developing a modern data platform is still in its early stages, we are taking these initial steps.
With our legacy systems, we have already successfully implemented over ten real-time use cases using our on-premise components without requiring any architectural changes to our core platform. This has enabled corporate banking to process checks in real-time, persist transactions instantly, handle payrolls, and offer other essential services. Moving forward, as we transition to the cloud, we will be deploying more real-time AI solutions, expanding our capabilities even further.
Real-time analytics often involve dealing with large volumes of data. How does Confluent’s data handling capabilities support FAB’s analytics requirements?
It’s important to remember that while real-time processing is valuable, it doesn’t necessarily mean that everything has to be in real-time. In fact, for analytics, micro-batching often suffices and can be more cost-effective.
The advantage of the Kafka architecture is its flexibility—you can have a balanced combination of real-time, near real-time, micro-batching, and batch processing to meet your company’s specific needs. Our analytics team plays a crucial role in determining which dashboards, insights, and data components should operate within each of these different timeframes. This approach ensures optimal efficiency and effectiveness in data processing.
What role does data integration and synchronization play in ensuring the accuracy and timeliness of the FAB’s real-time analytics and payment processing?
I would argue that it’s the most critical role in our organization. While stakeholders often focus on results like appealing dashboards, cutting-edge data science models, and advanced AI, these achievements are only possible with a well-structured, scalable, flexible, and data-governed platform.
To ensure this, we strongly emphasize managing our data pipelines with proper data governance, prioritizing data quality and integrity. Equally vital is the alignment of our data sources with the data platform, leveraging technologies such as schema registry with Kafka and employing data contracts within the Kafka layer. This holistic approach is the foundation of our success in delivering valuable insights and solutions to our stakeholders.
How does FAB manage data security and compliance when dealing with sensitive financial and customer data in real time?
Indeed, Kafka offers a fantastic feature in this regard. The management of data sensitivity is a top priority for all our platforms, and we uphold a stringent definition of Personally Identifiable Information (PII) across our systems. We consider almost every customer data point as PII, which is an exceptionally strict approach.
However, real-time processing adds an extra layer of complexity to this challenge. Deploying a data catalog or categorizing PII data in real-time can be quite demanding. Fortunately, with the help of Kafka and KSQL, we’ve been able to streamline this process. We’ve implemented various rules and even integrated Python scripts that assist us in categorizing data effectively. This ensures that only non-PII data is processed for analytical and data science purposes, while PII data is securely stored where it belongs.