Building a digital innovation team to leverage AI
Khaled AlShami, Infor vice president solution consulting, Middle East & Africa shares insights on Building high-performing AI/ML teams for successful digital transformation
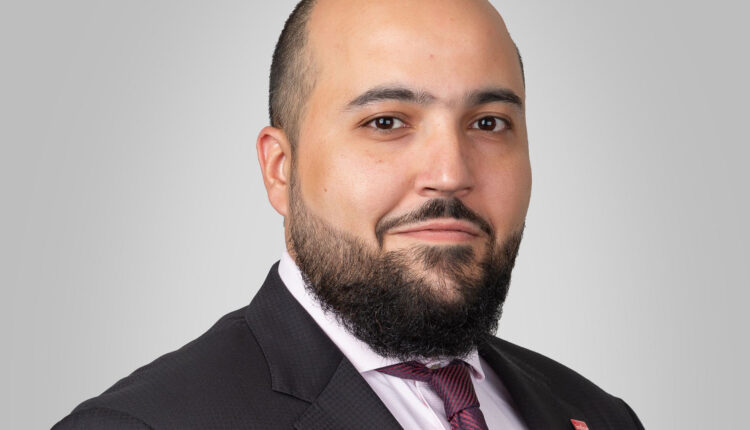
It seems like artificial intelligence is in every headline and news feed today. Companies looking to invest in digitising their business operations are inundated with various advanced techniques including artificial intelligence/machine learning (AI/ML) but do not have the necessary know-how or investment plans to succeed. Furthermore, the success rate for these investments is not guaranteed which impacts proper focus in the current economic climate.
The hype-hope-fear cycle creates two common scenarios in executives’ minds. A) deciding they are better off sticking to what works and ignore the noise, or B) reflexively deciding they need to get in on the innovation train.
The truth is it continues to be a challenge to successfully form and leverage high-performing AI/ML teams due to general misunderstandings regarding how to set up the organisation for success.
Figure 1: Circle of emotions businesses rotate through during digital innovation.
Data-driven solution roles
The most common reflex is to hire one or two data scientists with backgrounds in the latest cloud AI/ML solution and task an IT organisation to leverage the new role to kick off a digital transformation journey.
To build and adopt AI/ML solutions, look for the following roles to build your own in-house data solutions teams:
- A solution architect who understands how data flows between different systems. It is common to have 10 to 12 different ERP systems in different clouds and on-premise location in manufacturing organisations.
- A data engineer who can build and manage data pipelines and is able to understand big data strategies.
- A data scientist who can leverage existing models for business value. Data scientists specialise, so depending on if you are looking at manufacturing process improvement (scrap, yield) or supply chain visibility, you need to make sure they have the right skills.
- A research scientist if you are looking for something very specialised for your business. In the case where you are looking for prescriptive analytics, this role will have a specialised degree for production scheduling or inventory optimisation to name a few common use cases.
- A data storyteller who can support the communications between the business and the data science team.
- An AI/ML project manager with experience in agile data project management and tracking and communicating with IT and business.
- A ML operations person who is responsible for managing solutions that are in production.
It is common to combine these roles, but it is highly unlikely you will find one person who can do all of them properly.
Figure 2: Common minimal recommended roles for in-house data solutions team
It takes a village
The $1 million investment required is not hyperbole. Beyond the specialised data science team, it is important to have certain roles within an IT organisation that can support data/integration tasks, security and any reporting needs an organisation requires to support its digital strategy.
Internet of Things (IoT) based systems require specialised skills to maintain and manage sensor data including collection, processing and leveraging for use. With the focus on cyber challenges additional roles are required to manage data protection and privacy of sensor information. These roles are additional and complement the data solutions teams.
The output of AI/ML solutions drives business change, so it is important to invest in educating and training the shop floor operators, planners, and quality-control technicians on how to leverage and partner with the data science teams. The “learning” does not apply to machines only but also to workforces to achieve maximum ROI. For AI/ML solutions focused on supply chain, it is important to incorporate analytics to support sales, finance, and product management teams, so investment in analytics team and software is essential.
Very often, AI/ML solutions teams fail because of misunderstandings regarding how they can be leveraged and seamlessly integrated in the business.
Data-driven decision centre of excellence
The long-term advantage of leveraging data and AI/ML solutions is that it will dramatically improve your ability to maximise profits. Models to improve asset maintenance and spare parts will reduce costs of operations and improve safety. Creating solutions that monitor, alert, and recommend changes to manufacturing orders will reduce scrap and improve quality and customer satisfaction. Collecting information on product use and leveraging information on raw materials can improve product manufacturing and optimisation and improve revenue.
Other tangible business benefits include productivity improvements via automating manual processes. A combination of near real-time decision making and learning/teaching systems will reduce tedious tasks and allow new and existing workforce to remain productive without long on-boarding times.
To achieve these benefits, it is essential to create a centre of excellence-type model where the AI/ML team works with and for all business functions. This allows the team to learn and reduce attrition risk, since managing and updating the same solution is monotonous to the science team, as well.
Building teams and systems from scratch will take 12 to 18 months, and turnover is a significant risk in this market without the proper support in nurturing talent and careers.
Innovation starts at the top
For successful adoption of AI/ML, leadership needs to be educated on the total cost of ownership and pragmatic business outcomes. It is essential to hire an executive role, preferably as a business function, whose main charter is building innovation strategy, evangelisation and reporting using data-driven key performance indicators (KPIs) on performance of AI/ML initiatives. AI/ML solutions need to be quick and measurable based on business performance to prevent buyer’s remorse.
As businesses leverage data to drive business outcomes, it is also important to have an executive role in charge of managing, communicating, and prioritising the focus on the next AI/ML solution.